Resource wind
Use the wind effectively
The resource wind as “fuel” of wind energy determines the energy yield of a turbine and influences its lifetime.
ForWind conducts research to predict wind conditions for wind farms and regions as accurately as possible.
Wind potentials can be determined and expected power outputs derived from studies of large- and mesoscale weather development.
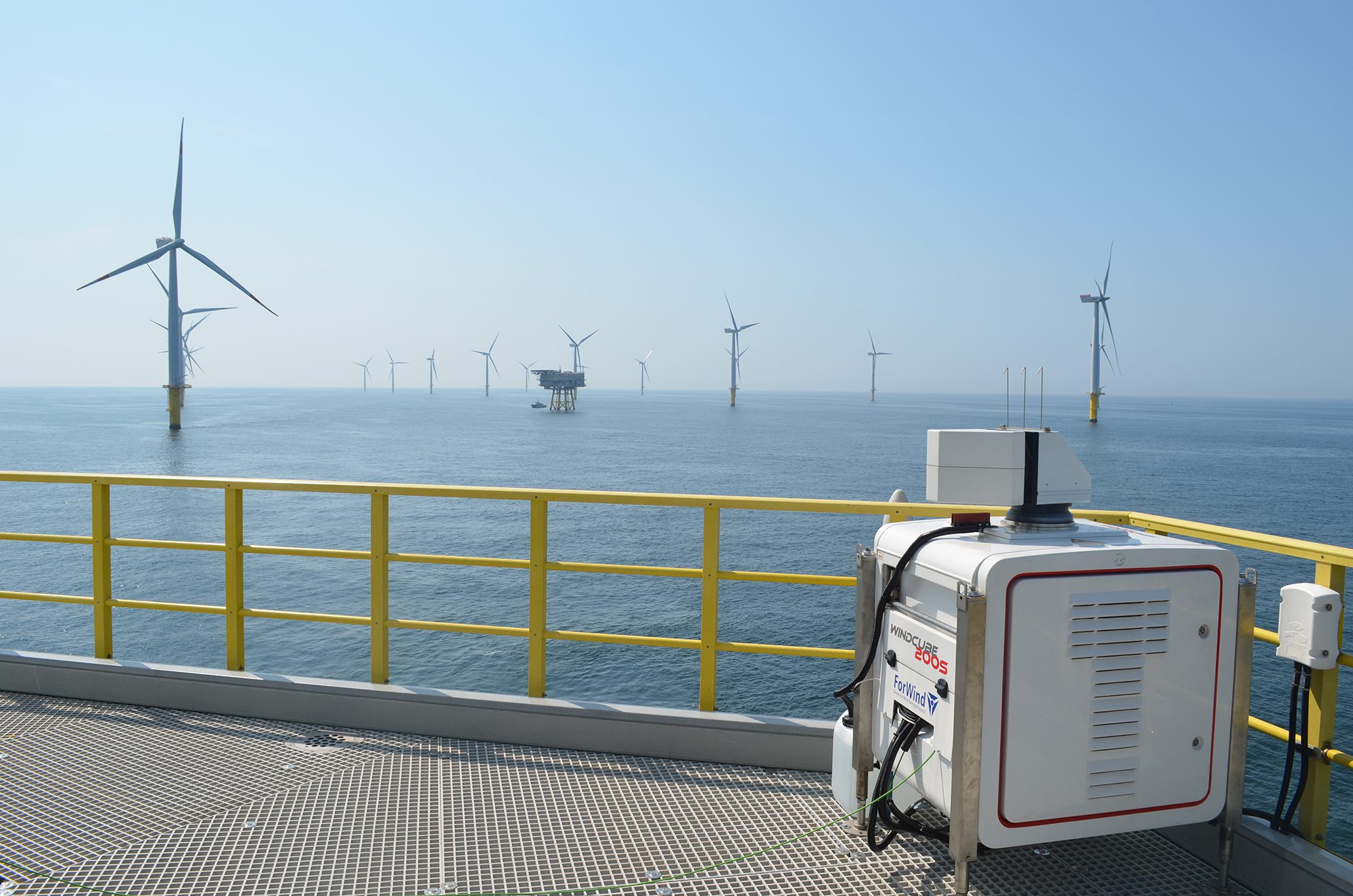
Lidar & Wakes
Lidar (abbreviation for “light detection and ranging”) is a remote sensing technique for measuring wind flow that has applications in both industry and wind energy research. The measurement method works in a similar way to the radar trap used in traffic control, except that here it is not the speed of the road user that is measured, but the speed of tiny particles and droplets that are in the air and transported by the wind. The wind industry mainly uses lidar technology to assess wind energy resources and determine wind speed when surveying the power curve of wind turbines.
For example, we use lidar devices to measure complex wind flows of an entire wind farm or in the immediate vicinity of individual wind turbines. Part of our work is also exploring advanced methods for reconstructing wind fields from lidar measurements. With the measurement results, the interaction between wind turbines and flow can be better understood. We then use this knowledge to increase the efficiency of wind turbines and wind farms. We also use our work with lidar to develop new methods, i.e., to explore new reconstruction approaches and how well we can use lidar to measure the “truth” (i.e., the actual conditions present in a wind field).
Control concepts for wind turbines
The dimensions and rated power of wind turbines have continuously increased in recent decades to reduce the cost of wind energy. One of the main obstacles to further increasing dimensions is the disproportionate increase in structural loads. The reduction of these loads is an important focus in our research activities in the field of wind turbine control.
Control strategies for load reduction are developed in two areas:
- Development of new control algorithms based on either individual control of rotor blade angle or reduction of rotor blade and tower vibration,
- Analysis and use of novel concepts such as smart blades with active flaps or louvers on the rotor blades and lidar-assisted control
Energy Meteorology
The ongoing research activities of the Energy Meteorology team focus on meteorological aspects of wind energy. The objective is to describe the spatiotemporal characteristics of wind fields at different size scales using the method of computational fluid dynamics. In addition to modeling wind resources for onshore and offshore locations using the mesoscale model WRF, the group’s work focuses on investigating the interaction of atmospheric flows and wind farms using the large-eddy simulation model PALM. A current research project is also working on the question of what possibilities there are to influence the wake of wind farms in the German Bight and to increase the impact of the wake on the energy yield in the German Bight as a whole.
To represent the interaction between a flow in the atmospheric boundary layer and a wind turbine in detail, the team coupled the aeroelastic code FAST with the large-eddy simulation model PALM.
With the help of these coupled models, information about the wind turbine at different atmospheric flows can be obtained. For example, the loads, moments, and power of the system can be studied as a function of boundary layer properties. Different turbine models were implemented, with the goal of reducing computation time as much as possible without loss of information.
Large-eddy simulations place very high demands on computing resources. Therefore, so far only single situations can be simulated with this method. However, these data form an essential basis for the further development of comparatively inexpensive methods for simulating the flow conditions at a site or the flow conditions in a wind farm. The Energy Meteorology team is currently involved in a research project to develop a method for deriving LES-quality information for flow conditions at a site from comparatively inexpensive mesoscale simulations and artificial intelligence methods based on them.
In addition to performing numerical flow simulations, the team also conducts its own micrometeorological measurements, e.g., with eddy covariance stations. The primary goal of these measurements is to validate the results of the flow simulation models.
Projects on the research topic “Resource Wind
X-Wakes research project
The main objective of the X-Wakes project (funded by BMWK) is to record the changes in wind conditions for the operation of offshore wind farm clusters during the large-scale expansion of offshore wind energy use. This is done both by an extensive measurement program with airborne and satellite-based measurements and by LiDAR measurements at various locations within the German Bight. The interactions of wind farm clusters with each other, which will also be investigated in great detail with the numerical tool of large-eddy simulation in addition to the measurements, will be implemented in industrial models and in a mesoscale weather model in order to enable a realistic yield forecast for future expansion scenarios with these.
YawDyn research project
The overall goal of the YawDyn project (funded by BWMK) is to reduce the energy production costs of offshore wind farms. For this purpose, two novel methods for wind direction tracking (yaw control) will be researched, validated and published, which serve to increase energy yield and reduce operating costs. Research focuses on the development and validation of dynamic wind direction tracking concepts. YawDyn is intended to contribute to risk minimization in the application of novel methods for aerodynamic wind farm control in the exploitation of offshore wind energy.
WindRamp research project
Wind ramps, i.e. rapid changes in the incoming wind speed, cause strong gradients in the feed-in power of offshore wind farms. The prediction of such wind ramps is subject to large uncertainties. This makes efficient operational network management more difficult. The Lidar remote sensing technique allows wind speeds to be measured several kilometers upstream. This allows wind ramps to be detected before they hit the wind farm. The objectives of the WindRamp project (funded by BMWK) are improved grid operation management and optimization of power trading processes by researching and testing an observer-based short term forecast of wind farm performance based on lidar wind measurements and the parallel concept development of a compact lidar with significantly increased measurement range.
Research focus
Anemometer
The most commonly used method in meteorology for measuring wind speeds is the cup anemometer or cup anemometer. However, with this sensor, it is not possible to resolve turbulent velocity variations larger than 1Hz due to its inertia. In addition, asymmetry in the response time to increasing and decreasing wind speeds causes the effect of overspeeding, which can lead to measurement errors of up to 10 percent. This is particularly problematic when determining the wind energy potential at a site, as this maximum error in wind speed can lead to an error in power prediction of up to 30 percent. This is because the wind speed enters cubically into the calculation of the power and thus ultimately into the calculation of the energy contained in the wind.
Corrections to the half-shell anemometer
Since the mid-1950s, correction methods have been developed by various authors to deal with the problem of overspeeding. Previous approaches require additional information about the geometry of the respective shell cross anemometer or about the wind direction for this purpose. ForWind treats the shell cross anemometer as a stochastic system with Markov properties. Here, the condition to which the system shell cross anemometer drifts is sought. First results show that the new approach provides promising results and the determined mean wind speed is closer to the actual mean wind speed than with the mentioned correction methods.
New development: The spherical anemometer
ForWind is also working on researching alternative measurement methods such as the spherical anemometer. Here, a ball is fixed to the end of a rigid tube, and the other end of the tube is fixed in a holder. If this tube-ball combination is in an air flow, the construction bends minimally depending on the wind speed. This small bending can be determined with the help of a light pointer, for example. Initial tests with the new sensor in the wind tunnel have delivered promising results.
Concepts for wind farm regulation
By converting some of the energy contained in the wind, the wake of a wind turbine is characterized by lower wind speeds and higher turbulence. This results in lower power generation in the rear turbines in a wind farm and higher loads due to turbulence. Modern wind farms typically use very simple control strategies that do not take into account this interaction between wind turbines. The development of a suitable control system could increase energy yield, reduce structural loads, and improve the integration of wind energy into power grids. Our main research objectives are:
- Develop wind farm and wake models that are simple enough to be used for controller design, but detailed enough to capture the relevant physical phenomena.
- Development of control algorithms that take into account the interaction between wind turbines and are able to respond dynamically to changes in the wind.
- Develop control algorithms suitable for various control objectives such as power maximization, active power control, and load reduction.
Performance predictions
Predictions for estimating the performance of wind farms are made at different time horizons using different methods. In a forecast range of several hours to days, forecasts are made using physical models such as numerical weather prediction models. In the range of only a few minutes to an hour, we speak of short term performance forecasts. These are becoming increasingly interesting for electricity trading and transmission system operators, but so far cannot be created with physical models. Such short-term forecasts are usually based on statistical models, which, however, are often unable to represent sudden fluctuations within very short periods of time well, if at all. Therefore, a new approach envisions making short-range performance forecasts based on real-time measurement data via lidar.
Installed on a wind turbine within an offshore wind farm, a lidar device can scan for several kilometers. This enables it to record the wind speed and direction of the wind field that will soon hit the wind farm. Based on this spatially and temporally highly resolved wind field information, it is calculated how much power the wind farm will produce when this wind field passes through it. The time horizon of the prediction depends on the range of the lidar and the magnitude of the wind speed. Suppose a lidar detects a wind field 10 kilometers upstream with a wind speed of 11 meters per second. The power forecast calculated from this would be available about 15 minutes in advance, before this wind field hits the first turbines in the wind farm. The results of our studies show that lidar and radar measurement systems can improve the accuracy of offshore wind power prediction. In particular, extreme and rapid changes in wind power due to abrupt changes in wind speed, e.g. during the passage of weather fronts, can be predicted better with this method than with statistical methods.
One focus of our current research is to extend lidar-based predictions into a methodology for observer-based probabilistic performance predictions for individual wind turbines and aggregate wind farm performance. For this purpose, lidar-based forecasts are combined with SCADA-based forecasts that predict wind vectors from wind turbine operational data. The combination of lidar and SCADA-based forecasts can significantly improve both the forecast quality and availability of a probabilistic 5-minute forecast for an offshore wind farm. These approaches will be further explored in future research projects.
Mesoscale modeling of wind resources
The development of wind energy requires more and more high quality information about wind resources in temporal scales from 10 min to several decades and in spatial scales from 10 m to 100 km. In addition to using available data from measurement stations, new sensor technology such as lidar, and other remote sensing techniques, atmospheric (mesoscale) flow models are used in particular.
ForWind uses the mesoscale model WRF (Weather Research and Forecasting Model, NCEP/NCAR) to investigate in particular the influences of the North and Baltic Seas and of complex terrain on the wind field. This model usually uses spatially and temporally coarsely resolved data from global models as input to describe the influence of small-scale local and regional conditions via “downscaling” procedures. WRF data can thus be used to estimate wind potentials at sites where measurements are not possible or are too time-consuming and costly, at any altitude.
In conjunction with long-term data (e.g., reanalysis data, climate scenarios), variability and extreme situations are also investigated on long-term time scales. Mesoscale model runs are flanked by simple parameterized models and by small-scale high-resolution models such as Large Eddy Simulation (LES).
Measurement of wakes of wind turbines and wind farms
When the wind flows through the rotor of a wind turbine, kinetic energy is extracted from it. This creates a wake behind the turbine that propagates downstream. In this flow, the wind speed is lower and the turbulence is greater than in the free flow. Plants located in this wake generate less energy and are subject to higher structural loads. Accurate characterization of wakes plays an important role in optimizing the design of planned wind farms. In existing wind farms, if the position and propagation of the wake are known, the wind farm performance can be optimized by clever control strategies. For this purpose, we investigate the dynamic behavior of the wakes and develop computer models to describe them.
For certain wind directions and speeds and dense spacing between turbines, wake losses can reach significant levels. In large wind farms where turbines are located in close proximity to each other, such wake losses often have a greater impact. However, an optimized layout for a typical offshore wind farm or wind farm cluster will ensure that overall wake losses are in the range of 10% or less of the potential annual energy yield.
Lagging effects from large wind farms and wind farm clusters can extend up to 100 km downstream. These cluster wakes can be very long-lived under certain atmospheric conditions. Often wakes recover within the first few kilometers downstream of the wind farm. Therefore, it is still difficult to calculate the overall impact of long-range cluster wake effects over the lifetime of a wind farm. Our measurements enable us to model the wake effects over scales of several hundred kilometers.
For the measurement of wake we place long range lidar devices on the nacelle or measure e.g. from the transition piece of a turbine the wake of a turbine or a whole park.
Measurement of the incoming wind at a wind turbine
In addition to studying wake effects, our research group also uses lidar devices to measure the incoming wind at wind turbines. To better understand the interaction between wind turbine and wind flow, we need to characterize the wind acting on the wind turbine. To measure the incoming wind field directly in front of a turbine, we use a short-range continuous-wave lidar. The “SpinnerLidar” is mounted in the rotating spinner or on the nacelle of the wind turbine and scans a circular section of the incoming wind field. In this section, the SpinnerLidar can measure every second at up to 500 different points. Thus, wind speed, wind direction, vertical wind shear as well as turbulence are recorded. Alternatively or additionally, WindScanners can be used, which are placed on the ground (near the turbine). The use of two instruments that can measure synchronous and flexible trajectories allows direct derivation of two-dimensional wind speeds. We match this wind data with the sensor data of the wind turbine. This allows us to see which wind characteristics have which effects on turbine behavior.
Large wind farms and wind farm clusters not only extract a large amount of energy from the incoming wind, but they also present considerable obstacles for the wind to pass through from the atmospheric flow. We investigate these effects using long-range scanning lidars installed at offshore wind farms to measure incoming wind fields. The cumulative effect of the buildup of wind flow is called the Global Blockage Effect. For a single wind turbine, this effect extends over a distance of about two to three rotor diameters from the turbine upwind. In the case of larger offshore wind farms and wind farm clusters, larger areas of reduced wind speed occur upstream of the turbines. The strength of the GBE depends on the size of the wind farm, its layout, turbine density, and atmospheric flow conditions – these parameters will be further investigated in future research projects. Compared to the wake effect, the global blockage effect is rather small, but should be taken into account when determining annual energy production.
Modeling of the relevant physical phenomena
In addition to simulating individual wind turbines and wind farms using numerical methods, we also carry out experimental work in the laboratory and in the open field. This not only supports the work in modeling and controller development, but also improves the understanding of the underlying physical laws. The focus of our experimental activities is on the interaction of wind turbines and wind farms with a realistic turbulent inflow, covering topics such as dynamic inflow, aeroelastic phenomena, and wake modeling, among others. For the experiments in the WindLab wind tunnel, we use scaled and controllable models of wind turbines of different sizes as well as a segment of a rotor blade with an active flap at the trailing edge. The generation of the dynamic incident flow as well as the measurement technology used correspond to the current state of science and research. For validation, we also use measurement data from tests in the free field.
Stochastic wind field modeling
Wind turbines use wind in the atmospheric boundary layer as a resource. Unlike hydropower, this resource is highly transient, exhibiting extreme fluctuations in space and time on small scales. Understanding wind fields is therefore of great importance for the correct dimensioning of wind turbines.
The goal of stochastic wind field modeling is to analyze and extend existing wind field models. Currently, conventional approaches are mostly based on spectral methods. The central criticism of this is that all statistics are Gaussian in nature, in contrast to experimental results for atmospheric flows that predict an increased occurrence of extreme small-scale wind gusts.
To improve the modeling of the intermittent dynamics of atmospheric turbulence, ForWind therefore uses current approaches from the dynamics of complex systems and turbulent flows. On the one hand, Continuous Time Random Walks (CTRWs), a special class of random processes, are used. These could be identified in the context of Lagrangian particle trajectories in turbulence theory. Another approach is based on stochastic modeling of the turbulent cascade over many size scales based on recent results of research in Oldenburg. This method is capable of reproducing, in principle, arbitrary multi-point correlations of turbulent flows in addition to two-point correlations. Furthermore, the method allows to exactly integrate real experimental measurement data of such superstatistic wind fields into a realization of a wind field and thus to transform incomplete free field measurements of e.g. meteorological measuring masts, lidar, or drones into a high-resolution wind field.
Scientists with research focus on wind as a resource
We do research!
Prof. Dr. Martin Kühn
University of Oldenburg - Institute of Physics
Wind Energy Systems
Tel: +49 (0)441 / 798-5061
Fax: +49 (0)441 / 798-5099
Email: wesys.office@uol.de
Prof. Dr.-Ing. Andreas Reuter
Leibniz Universität Hannover - Institute for Wind Energy Systems
Institute Management
Tel: +49 (0)511 / 762-4712
E-mail: andreas.reuter@iwes.uni-hannover.de